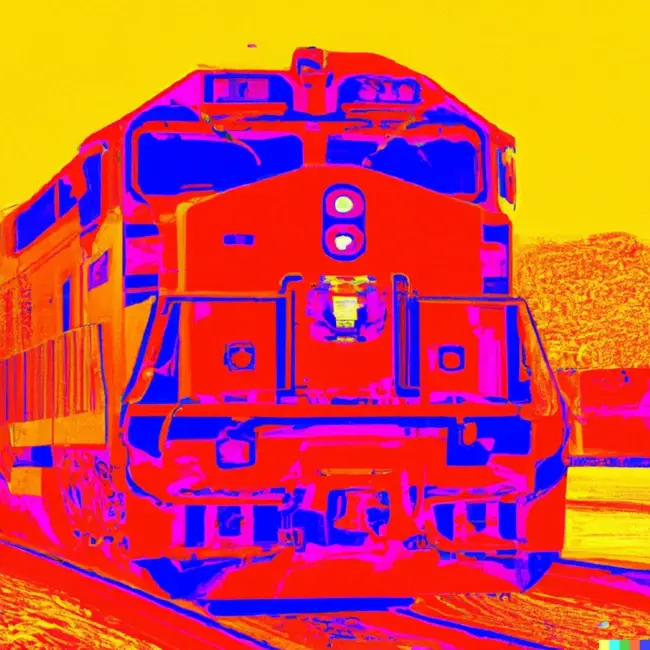
The following is an excerpt from the book, "Data Juice: 101 Real-World Stories of How Organizations Are Squeezing Value From Available Data Assets."
DB Cargo, the management company for Deutsche Bahn’s Rail Freight Business Unit, faces a serious logistics challenge of transporting at least 300 million tons of cargo every year across Europe and Asia. To drive efficiency across its operation, DB Cargo turned to Splunk Enterprise to handle the large volume of diverse data in real-time, providing real-time insights across fleet control, operations, maintenance, and engineering. Splunk alerts are tied to a rules engine based on failure code tables, enabling the locomotive team to decide the best action to take when a failure occurs.
By analyzing the data coming from many sources, DB Cargo has been able to keep locomotives in service longer, reduce maintenance costs, and ultimately deliver better service to its customers, making DB Cargo more competitive. The locomotive manufacturers can also use the data provided to identify occasions when locomotives can stay in service longer and recommend whether the locomotive needs to go to the maintenance workshop or not.
DB Cargo’s real-time approach to visibility into the data and tying that data to action via a business rules engine provides direction to the locomotive team. This approach applies to industries that deal with large, expensive, and complex machinery, such as mining, construction, shipping, and transportation. Rules engines are effective but reactive and based on expert knowledge. A more proactive approach would augment rules with machine learning that predicts future failures well before they occur.
However, there are challenges such as sensors and log files not always telling the whole story, limits of legacy old-school technology’s ability to log critical data, and the need to assess what data is most useful to solve problems. Simplifying the problem by piloting collecting many streams of information on a few locomotives, experimenting on what predicts outcomes, and finally rolling out widespread data collection based on what has proven utility can help.
DB Cargo’s wealth of data will also be useful in optimizing capital investment decisions. Collecting detailed insights on locomotives’ actual performance in different weather, humidity, air quality, and track conditions over time will enable DB to better procure and deploy equipment based on its best use. DB will also develop detailed insights on supplier and manufacturer quality, potentially marketable back to manufacturers or non-competitors in the same industry and prove useful in procurement decisions and negotiations.
DB Cargo’s approach to real-time analytics of locomotive data has enabled the company to optimize locomotive operations, reduce costs, and deliver better service to customers. The challenges in analyzing data from legacy technology, human input, and determining the most useful data can be overcome by adopting a proactive approach that leverages machine learning and simplifies the data collection process. DB Cargo can also optimize capital investment decisions by analyzing locomotive performance data and supplier and manufacturer quality.