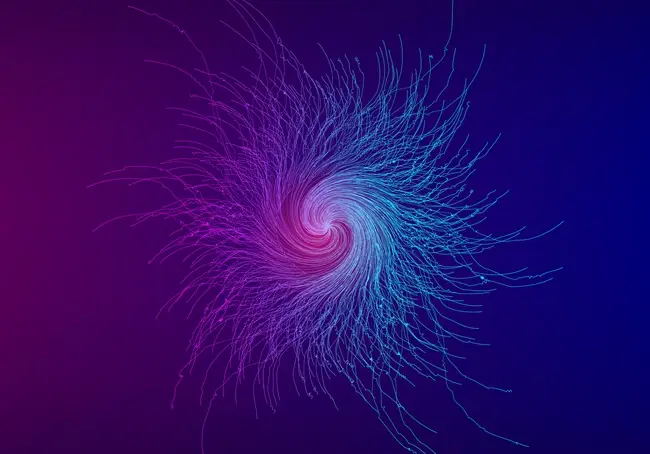
Data Mesh vs. Data Fabric: Comparing the Paradigms
Centralization vs. Decentralization
Data Fabric represents a centralized, technology-driven approach aimed at creating a unified platform for managing and accessing data across various sources. It focuses on automating data integration, providing a consistent data infrastructure that leverages AI and metadata-driven architectures to enable real-time analytics. By offering an abstraction layer, Data Fabric hides the complexity of diverse data sources, making seamless data access possible.
In contrast, Data Mesh decentralizes data management and ownership. Here, individual teams or business units take responsibility for their own data, creating "data products" for internal use and potentially for others within the organization. A data mesh ensures that data is accessible, discoverable, and usable across the organization, while federated governance enforces standards to maintain data quality and usability.
The diagram below illustrates the main components of each approach. It shows fabric’s technical components that provide a unified platform from data sources to data consumers, while data mesh consists of a set of decentralizing organizational and operational concepts emphasizing domain autonomy.
Advantages and Disadvantages
Their differences in approach come with different advantages and disadvantages. The table below outlines the trade-offs between the two paradigms.
Blending Mesh and Fabric: A Solution Spectrum
With an understanding of the trade-offs that mesh and fabric entail, organizations need to find the right balance between central control and decentralized autonomy. This balance, driven by their needs, data maturity, and business model, results in a unique blend of elements from both.
It’s helpful to frame data mesh and data fabric as part of a solution spectrum. On one end is data mesh with its independent functions, such as defining domain data terms and meaning and ensuring data assets are relevant and reliable to the business. On the other end is data fabric with its shared functions, such as data access control and a common interface for finding and consuming data assets.
Determining Your Organization’s Place on the Spectrum
This mental model offers organizations a framework to strategically blend the benefits of control and autonomy. Finding the right place on the mesh-fabric spectrum depends on several factors:
Regulatory and Compliance Requirements. Highly regulated industries, like finance and healthcare, require strong shared governance functions to ensure compliance and data security. Organizations in these sectors may emphasize centralized control to enforce consistent standards while allowing some domain autonomy for specific business needs.
Cultural Readiness. Organizations with a culture that values autonomy, ownership, and collaboration will find it easier to adopt independent domain-level functions associated with data mesh. Companies that prioritize top-down decision-making often resist incorporating independent functions that enable domain agility making data fabric a more natural fit.
Data Maturity. Organizations with mature data governance frameworks can be better positioned for independent domain-level functions, allowing domain experts to own and manage data assets. Less mature organizations may need stronger centralized controls to ensure quality, consistency, and scalability as they build their data capabilities.
Business Agility. Fast-moving industries, like tech or ecommerce, require greater domain autonomy to enable rapid innovation. These organizations can adopt decentralized data management to align more closely with dynamic business needs while maintaining some centralized functions to support discoverability and cross-domain data usage.
Scale and Complexity. Large organizations with complex data environments may need to rely on centralized functions for standardizing key data practices across business units, such as defining data quality benchmarks or ensuring access to shared infrastructure. At the same time, they will need independent domain-specific functions to ensure relevance and responsiveness to individual business units.
Data Products: A Common Thread
Once a company determines how it needs to define an optimal balance of control and autonomy, it needs a practical way to implement it. Enter data products. The concept of a data product ties data mesh and data fabric together. A data product is a data asset that has all the characteristics of something that can be consumed by people you don’t know. It must be standardized, packaged, shoppable, deliverable, and returnable. Creating data products that meet this standard requires both shared and independent functions.
Shared Functions of Data Products
The following shared functions ensure that while each domain operates independently, their data products remain aligned with the broader organizational standards and practices, ensuring consistency and trust:
Data Quality Standards. Central teams define and enforce organization-wide data quality benchmarks to ensure that all data products meet a minimum threshold of reliability.
Data Discoverability. Centralized metadata management and data catalogs make data products accessible and discoverable across the organization, preventing data silos.
Security and Compliance. A shared governance enforcement layer ensures that all data products adhere to security protocols, privacy regulations, and compliance standards.
Interoperability. Centralized governance enforces data interoperability standards, allowing seamless integration and use of data products across domains.
Data storage. Domains get carve-outs in an enterprise data platform where they can mix and match their own data with enterprise data to build products without impacting other domains.
Independent Functions of Data Products
The following independent functions allow business domains to innovate and make faster decisions:
Domain-Specific Data Terms and Models. Each domain defines its own data terms, schemas, and models, ensuring that the data products are tailored to their specific business needs.
Data Relevance and Context. Domain teams curate data products to ensure they remain relevant and aligned with the fast-changing demands of their respective areas, enabling more accurate decision-making.
Local Data Operations. Domains control how they process, clean, transform, and store data, giving them the autonomy to iterate quickly and adapt to business changes without relying on central teams.
The Data Marketplace: Where the Balancing Act Happens
A data marketplace is where organizations can implement their balance of both shared and independent functions. It allows users to browse, acquire, and consume data products from different domains with rich metadata that allows them to understand, evaluate, and access them for their business needs.
The marketplace enables shared governance by providing standardized tools for data discoverability, quality control, and data access control, ensuring that all data products meet organizational standards. It empowers individual domains by allowing them to create, manage, and publish data products tailored to their specific business needs but that may also be useful to other domains.
This diagram illustrates how the data marketplace is part of a common data platform that enables domains to create data products managed by shared data standards.
Establish a Foundation
No matter where you land on the solution spectrum, it’s critical to establish a solid foundation for future growth. Key foundational elements include:
Governance: A strong governance framework, whether centralized or federated, ensures data quality, security, and compliance across the organization.
Metadata Management: For both fabric and mesh, metadata plays a vital role in making data discoverable and interoperable.
Data Culture: Fostering a culture that values data as a product and encourages collaboration across teams is essential to success in both paradigms.
Building an Adaptive Data Ecosystem for Long-Term Success
As data management evolves, the balance between centralized control and decentralized autonomy will continue to shape new opportunities for innovation. The future of data strategy lies in adaptability—the ability to shift dynamically as business needs, technology, and data complexity advance. By embracing a hybrid approach that combines elements of both Data Mesh and Data Fabric, organizations can create scalable, agile, and intelligent data systems that empower decision-making at every level. Those that view data strategy as a flexible framework, rather than a fixed choice, will be well-positioned to thrive in an increasingly data-driven world.