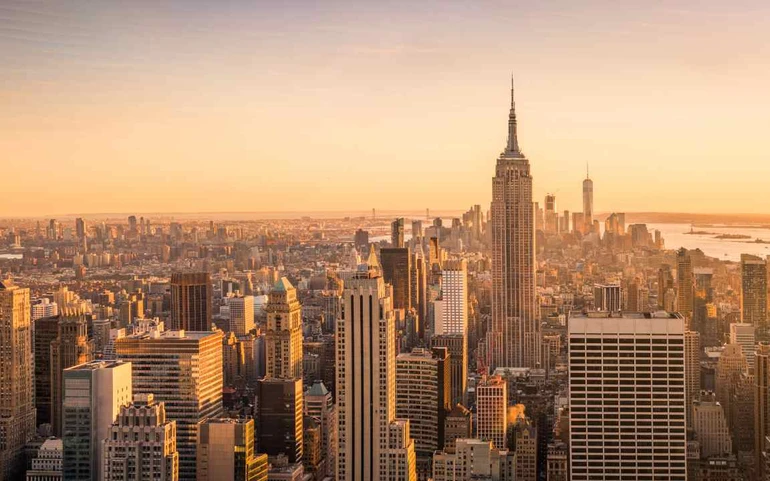
By Sara Faatz, Director, Technology Community Relations, Progress
As digital experiences play an increasingly central role in our lives, organisations in all sectors realise they must embrace new technologies to meet ever-changing consumer needs. Generative AI is one game changing technology that’s capable of elevating digital experiences, making them more engaging, personalised and simplified.
This potential influence of AI is so great that Gartner predicts by 2025, almost a third (30%) of outbound marketing messages from large organisations will be synthetically generated, up from less than 2% in 2022. Another likely scenario is that by 2030, a major blockbuster film will be 90% generated by AI (from text to video), from 0% in 2022.
But every silver lining has a cloud - and to reap the benefits of generative AI, enterprises can face a multitude of technical and ethical challenges when attempting to integrate it. There are five areas that organisations must be cognizant of in order to integrate generative AI well, and to deliver next-level digital experiences.
Effectively integrating generative AI
Here are some considerations for effective integration, from mitigating the risks of unconscious bias, to security, privacy and other technical considerations:
Training patterns and unconscious biases
A critical success factor in generative AI is the quality of content-generating models. Tech teams must carefully curate the training data of this content, and it must represent the diversity of the entire user base and use cases that generative AI will encounter.
Failing to do this runs the risk of allowing unconscious bias to creep in - a significant problem in generative AI because these systems are only as good as their training data. Teams can detect and mitigate bias through automated tools and technology. By taking a proactive automated approach, organisations will ensure their generative AI systems create fair, inclusive and engaging digital experiences.
Hyper automation
Combined with generative AI, hyper automation has the potential to revolutionise the way companies create and deliver digital experiences. Automating core processes can save critical time, reduce errors, and allow skilled employees to be focus on higher-level tasks. It can also create more personalised and engaging customer experiences.
However, it requires organisations to have the human expertise and deep knowledge of how these technologies work and can be integrated into existing systems. One solution is to leverage automation to streamline processes and use generative AI to create personalised and engaging content. In this way, the full potential of hyper automation is harnessed to create efficient, human-centric digital experiences.
Security and privacy risks
A major challenge in securing generative AI is protecting the underlying datasets. Since it relies on large data sets and complex algorithms, generative AI is easily susceptible to various cyber threats and malicious actors.
Security teams must establish robust access controls and authentication techniques to protect datasets used in generative AI training, which may include encryption, multi-factor authentication, and function-based access controls. The generative AI system can also be monitored and audited to detect potential security threats. Beyond technical measures, data privacy and compliance are critical for generative AI.
Scalability and performance
Since considerations for scalability and performance are vital, distributed computing and cloud computing approaches help enterprises to grow and scale successfully. Distributed computing is a way to improve performance and minimise latency, allowing tasks to be divided into smaller, more manageable segments that can be processed simultaneously on multiple nodes, ensuring consistent and accurate output.
Additionally, cloud computing services provide virtually unlimited resources that can be flexibly allocated to accommodate fluctuating demands. Continuous monitoring of performance metrics also helps to identify potential bottlenecks and areas for improvement.
Technical considerations for implementation
When integrating generative AI into digital experiences, it is important to select the appropriate type of generative AI for the specific use case, as each type has its strengths and limitations.
Some best practices to bear in mind include fine-tuning use cases and identifying the specific business problem; thoroughly analyse existing datasets to identify biases or data gaps; select the appropriate generative AI type for a particular use case, ensuring compatibility with existing systems and workflows; create a detailed plan for training, testing and monitoring generative AI system performance, along with robust security measures.