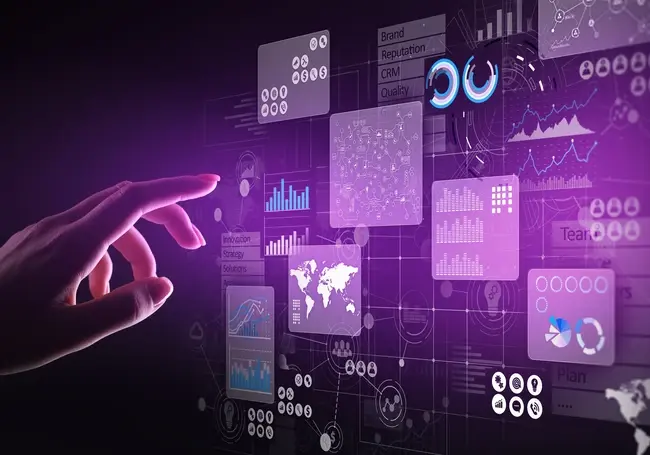
Implementing data products can enhance how organizations use data, but there are potential pitfalls. This checklist aims to highlight common missteps organizations make when deploying data products for business users. These pitfalls don’t apply to every data product: they pertain to coarse-grained data solutions intended for use by large numbers of business users or external customers. By understanding and addressing these pitfalls, organizations can successfully implement data products that drive business value.
1. Not Educating Executives about Program Management
Executives need to understand that data products require program management, not product management, and long-term, continuous funding. A data product is a strategic commitment by the company to serve the needs of a target audience on a long-term basis. A data product is not delivered as a singular project with a one-time price tag; it requires sustained investment and resources throughout its lifecycle. Executives need to treat data products like commercial products where they commit funding to support a long-term program of product enhancement and delivery.
2. Appointing Inexperienced Product Managers
Experienced product managers are essential for guiding the development and delivery of data products. Not every data leader or manager is suited to the task. Product managers set strategic direction, establish goals and metrics, prioritize requests, create processes, estimate value and returns, create course adjustments, and calculate the ROI. They work closely with relationship managers and developers to define, build, and deploy data products and continuously enhance them. They sit at the nexus between executives, customers, and developers and balance their needs. This is not an easy job and requires individuals with prior product management experience to succeed.
3. Not Investing in Customer Service
A key part of a product management function is to maintain relationships with target customers. This relationship management or customer service function is critical to defining and enhancing data products. They consolidate requests, gather requirements, help create specifications for developers, and provide customer training and support. Relationship managers may not exist as a dedicated function, but their work is critical to the success of the team, according to Henrik Strandberg, author of “Data Product Management: Leveraging Data Products to Transition from Cost Center to Value Creator.” They provide a single point of contact for customers and enable product managers and developers to focus on their core duties.
4. Not Establishing a Process to Prioritize Customer Requests
The fundamental requirement of a data product is that it addresses a well-understood problem of a target customer and offers a considerable return on investment. Most data leaders are besieged with requests from business users for data solutions. It’s imperative that the product managers establish a process for evaluating and prioritizing these requests before investing time and energy designing and building a solution. If business users can’t adequately describe the problem and the business value the product will generate, and they can’t commit to implementing the solution, then the product manager needs to walk away from the request or have the requestor modify it to meet these requirements.
5. Inadequate Handling of a Data Product Backlog
There is nothing worse for business users to submit a product request and feel like it’s disappeared into the black hole of the data team never to see the light of day. It’s imperative for data teams to log all requests and provide estimated dates for both addressing and resolving the request. Business users should be able to check the status of their requests online. It’s also critical that all requests get triaged and placed in the appropriate review bucket. Small requests that come in via ticketing systems or email should be funneled to the appropriate developer. Larger requests from a project management office or high-demand department (e.g., marketing) should go through a formal review process.
6. Inadequate Data Infrastructure and Engineering
Without sufficient data infrastructure and engineering capabilities, a product team can’t execute on its vision. Either the initial development will take too long and cost too much or it will get bogged down addressing technical debt or systems integration work. Ideally, a data product team can leverage a cloud-based data environment that has well-defined zones of data processing that make it easy to build on past data structures and models to deliver new solutions. Ideally, the environment runs in one cloud platform and one data management system to simplify the process.
7. Insufficient Attention to Creating a Product Mindset
The biggest challenge organizations face when implementing data products is “creating a product mindset.” This was mentioned by 70% of respondents in a recent Eckerson Group survey on data products. (See “The Data Product Revolution: A Market Study in Adoption Trends”.) A product mindset implies a consistent application of product management principles contained in this article. Executives make a long-term commitment to funding data products; the data teams hire product managers and relationship managers to understand and support customers; and the product team applies appropriate governance throughout the data product lifecycle, among other things.
8. Insufficient Product Metadata
Metadata provides essential context about data products, facilitating efficient usage and management. Overlooking metadata makes it hard for business users to find, evaluate, and use data products and for technical developers to troubleshoot problems and evaluate downstream impacts of their fixes. A data product contains three tranches of metadata: 1) metadata associated with the properties of the underlying asset(s), such as date created, developer, size, schema, sources, etc.; metadata from the data catalog where the assets are indexed, including lineage, owner, and related assets; and metadata from the data marketplace where the product is published, including terms of usage, terms of service, delivery channels, an subscription details.
9. Neglecting Data Governance
Most organizations implement customer-centric data products to increase the reliability and trustworthiness of data. But just calling a data asset a “data product” doesn’t change the underlying quality of the asset. To do that, data teams need to adhere to rigorous governance processes both when defining the data product and building it. (See “Data Product Governance” in “How to Create, Govern, and Manage Data Products” Market Landscape report, January, 2024.) This involves establishing both a product review board to review product definitions, value, and risk and a technical review board to evaluate the product’s scalability, performance, and adherence to business, technical, and regulatory standards.
10. Not Creating Service Level Contracts
Many people say the unique characteristic of a data product is its guarantee. That is, the product comes with a contract that obliges the data producer to deliver a data product with a certifiable level of accuracy, quality, and freshness, among other things. Contracts can be traditional service level agreements (SLAs) or YAML-based data contracts that can be enforced in code. Another form of contract, however, binds the data consumer to appropriate uses of the data product, such as when, where, and how long they can use the product. For example, there may be a license that prohibits a data consumer from using the product for more than 3 months or in a commercial product.
11. Not Implementing a Data Marketplace
A data marketplace fulfills the promise of data product. Although data products can exist outside of a data marketplace, once an organization amasses a dozen or more products, it makes sense to centralize them in an internal data marketplace. The goal of a data marketplace is to make it easier for data producers to create, publish, and monitor data products and for data consumers to find, evaluate, and consume those products. A data marketplace also manages subscriptions, defines access, automates deliveries, and sets terms of service and usage, among other things. A data marketplace is also a critical component in a data mesh.
12. Not Measuring the Value of Data Products
Failing to both estimate and measure the value of data products makes it harder to justify both initial and subsequent investments. To speak the language of business, Henrik Strandberg recommends several approaches, including Intrinsic Value Investment (IVI), Business Value Investment, and Performance Value Investment. The IVI calculates the value of data from a cost or revenue perspective, while BVI multiples that factor by the value of the domain in which the data is used. PVI, in contrast, focuses on the downstream impacts of the data if delivered properly or not. (See “Data Product Management: Leveraging Data Products to Transition from Cost Center to Value Creator.” The technique your organization uses will be determined by its internal financial standards and requirements.
Conclusion
Implementing data products requires organizations to navigate and mitigate various potential pitfalls. Since data teams have little to no experience with product management, it pays to bring in experienced product managers and pair them with experienced data managers to combine the best of both worlds. By addressing the challenges proactively and adopting best practices, organizations can enhance the success and adoption of their data product initiatives, driving innovation and delivering value to stakeholders.
Comments ( 0 )