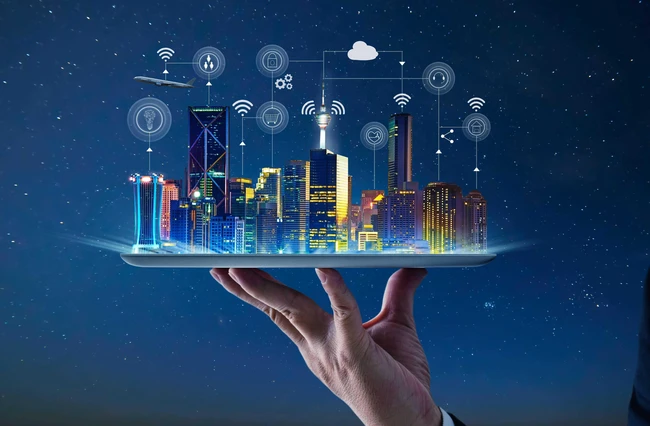
This article was contributed by Andrew Duncan, Partner and UK Head, Infosys Consulting
In a number of discussions that I’ve had over the last few months with company leaders, it’s clear that the global business world is fast evolving into something nobody could have anticipated before COVID-19. This article is the first in my three-part series on the future of work, and looks at the importance of building intelligence-based capabilities to help us prepare for, sense and respond to disruption. In the same way that we had to reconstruct our financial and risk models after the 2008 economic crisis, organisations today will have to reassess their use of AI and analytics to ensure our businesses are resilient enough to withstand further shock from future black swan events.
Better data and models
Our ability to collect, organise, analyse and react to data will be the new differentiator in business. The radical changes in professional, personal and societal routines have resulted in unprecedented shifts in consumer behavior. As a result, the historical data that fed many of our analytical models has quickly become out of date, incomplete and unsound. Organisations will look to conduct data and model audits to identify errors and weaknesses in operational, financial and risk areas. Underpinning this will be a new emphasis on data governance. We must take a forensic approach to real-time data capture across all forms and formats, both internally and externally. I also expect to see a new cloud-first business model become the standard. Cloud computing allows for data to be verified and stored securely, while still being available across multiple zones for deep analysis and insights.
Analytics led operations
Before COVID-19, there was a frequent disconnect between an organisation’s analytics and its strategic priorities. Now, even non-digitally native companies must put analytics at the very core of their operations. Accurate and timely data will form the backbone of all business units, from sales and performance forecasts, to procurement and supply chain optimisation. To achieve this, organisations will have to develop an analytics-led culture. This means not only including analytics leaders in all strategic discussions, but also embedding data-driven decision making from the ground up. I’ve seen first-hand how COVID-19 has created flatter, more agile organisations with empowered frontline employees. These employees should be given the analytical tools they need to make autonomous and informed decisions.
Digital twins
Businesses able to seize the opportunities created by digital twins can improve their predictive powers while reducing cost of service. As we emerge from the pandemic, more organisations will therefore turn to digital twins of their supply chains to better prepare for unexpected shocks and to build an intelligent and resilient ecosystem. However, with the increase of real-time data from rapid digitisation, I expect to see digital twins used not just in supply chains and manufacturing, but throughout all modern businesses. Using these models, we can experiment with a number of key variables, testing different scenarios and contingencies to proactively mitigate risks and extend capabilities across R&D, engineering, product management, and even sales and marketing.
Consumer focused automation
The surge in consumer call volumes arising from COVID-19 highlights the limitations of focusing only on human interaction in customer support systems. Post-pandemic, we will inevitably see a rise in automation in this area, with tools like voice biometrics and natural language speech recognition freeing up agent time and improving customers’ ability to self-serve. Analytics will also play a key role in enhancing the customer experience. By collecting data from a wide range of touchpoints, companies can gain a 360-degree view of their engagement levels. In my opinion, this is where AI is transformational, helping to pinpoint customers who are likely to leave or those need additional support, and finding the best offers to retain or assist them.
Synthetic data
When faced with black swan events, even the most well-honed and calibrated models quickly lose their predictive power. For example, financial models that use time-series, oil-price, or unemployment data will need to be rebuilt entirely. Several organisations are looking to increase the quantity and diversity of data available by using machine learning techniques to generate ‘synthetic’ data for model development. These vast sets of realistic data can be used to calculate risk measures, train predictive systems and stress test portfolios. Much of this work is still at the research stage, but I expect to see a growing interest in this area as we grapple with the impact of unprecedented events like COVID-19.
It is evident that this crisis has forced business leaders to reassess their analytics capabilities and accelerate their journey to digitisation. In the world ahead, I see business leaders, including myself, embedding data driven decision making into all levels of our organisations to gain greater intelligence and resilience.