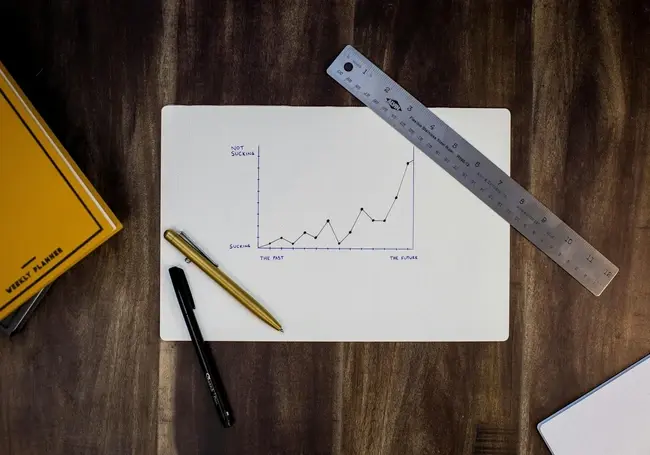
Healthcare businesses can leverage modern analytics to improve diagnosis and treatment strategies.
This approach will accelerate patients’ recovery and help medical researchers ethically acquire more insights into infectious and rare disorders.
However, many real-world integration obstacles limit the potential of insight extraction and reporting tools in this industry. This post will elaborate on the challenges and opportunities in implementing data analytics in healthcare.
What is Healthcare Analytics?
Healthcare data analytics develops and uses statistical models, machine learning (ML), and computer-aided visualization methods for clinical use cases. Analysts might customize those models to process clinical trial datasets. They can also modernize healthcare reporting and research practices.
Each stakeholder will require unique approaches to analytical models. For example, pharmaceutical firms will use data analytics solutions for the healthcare industry to test and improve drug formulations. Meanwhile, insurance companies will use them to estimate individuals’ health-related risks or prevent health insurance fraud.
The benefits of extracted health and life science insights range from flexible report designs to immediate data validation. Therefore, healthcare professionals can enhance communication between practitioners, researchers, and patients.
Importance of Healthcare Analytics
Aside from finding effective recovery methods, healthcare analytics enables hospitals, laboratories, and pharmaceutical businesses to streamline business development. Using optimized analytical models, stakeholders might discover market insights concerning registered patents, industry best practices, and competitor mergers.
At the same time, the rise of data governance regulations indicates an urgent need for privacy-friendly, ethical data management in health and life sciences. Consider HIPAA. It governs health insurance data portability and usage accountability. You will want to comply with it since it is a law outlining acceptable and punishable use cases involving patients’ electronic health records.
Finally, telemedicine and non-clinical lifestyle monitoring applications have gained momentum worldwide. Stakeholders are more willing to invest in robotic surgery techniques and innovative treatment interventions. Therefore, organizations require continuous market research in healthcare to track how these trends evolve.
Modern healthcare analytics assists in competitor research, governance compliance, market surveys, and industry trends reporting. Unsurprisingly, global institutions and business leaders in all markets want to employ the best analysts for excellent healthcare infrastructure development.
Challenges in Implementing Data Analytics in Healthcare
1. Data Quality Problems
Electronic health records (EHR) rely on human intervention to update patients’ databases. Human errors can adversely affect their data quality. Likewise, machine errors based on compatibility and portability might increase operational costs while hurting data integrity.
A robust healthcare analytics system must overcome human errors and technological hindrances for reliable integration. After all, poor data quality will skew insights, misguiding healthcare professionals. They will get undesirable patient outcomes if they use those insights to optimize treatment strategies.
2. Regional Law Variations
Policymakers in every country have enforced distinct frameworks to determine the role of patients' consent in handling sensitive medical details. They also offer guidelines concerning data localization and transfer for advanced research projects.
When a healthcare business enters new markets, it must inspect the increased legal risks due to varying legal requirements between nations. You want to leverage health and life sciences analytics without attracting non-compliance penalties.
3. IT Infrastructure Constraints
Current computing devices at a healthcare institution might lack resources for comprehensive analytics and market research projects. Organizations must consider financial feasibility and gather investors’ support to procure new equipment. Otherwise, they will implement standard analytics features, slowing digital transformation and losing the competitive edge.
So, the unavailability of technological assets is one of the expensive challenges in implementing data analytics in healthcare. Migrating to a governance-friendly cloud computing platform can help you tackle this challenge.
4. Cybercriminal Activities
If malicious parties access a patient’s clinical history records, they might threaten the individual by forcing them to pay ransom. After all, publicized EHR insights can become an instrument for damaging a person’s social, professional, and psychological well-being due to cybercrimes.
Therefore, each healthcare enterprise must identify and mitigate data theft and ransomware risks to safeguard stakeholders’ medical reports. End-to-end encryption and multi-factor authentication are suitable measures to contain data leak threats.
Opportunities in Healthcare Data Analytics
1. Population Health Management System and Policymaking
A population health management system (PHMS) can document a community’s health dynamics, lifestyle quality, health insurance coverage, recurring epidemics, and reliance on medicines. It will help regulators, hospitals, pharmaceutical companies, and laboratories across inventory and demand projections.
Furthermore, a PHM system can improve policymaking in high-alert situations like pandemics where individual-centric or regional strategies are ineffective.
2. Medical Personalization and Equipment Adjustments
Patients require specific treatment strategies due to allergies, financial concerns, and age-based weaknesses in natural recovery processes. Therefore, clinicians and pharmacists must use health and life sciences analytics to personalize prescriptions.
Similarly, each patient might request customized wheelchairs, lenses, braces, and implants for comfortable movement or better utility. Health equipment manufacturers can experiment with many design ideas using analytics to facilitate patient-friendly accessibility products.
3. Ease of Controlled Access and Collaborative Data Sharing
Hospitals and clinics often refer patients to independent medical specialists or laboratories. Modern healthcare analytics helps streamline data sharing among these entities. Besides, the host can restrict an external party’s usage and modification rights to protect EHR’s data integrity.
Version control tools and changelogs also make diagnosis monitoring more manageable. You can use them to quickly find who is responsible for a specific decision for investigative purposes.
4. Staff and Inventory Management
Public and private healthcare facilities have witnessed several challenges due to a lack of skilled workforce amid a global pandemic. However, analytical models can assist organizations in planning human resources for resilience against sudden peaks in cases during infectious disease outbreaks.
Each institution also wants to ensure sufficient blood bank, chemical inventory, and medical equipment stock. Data specialists can use ML-powered analytical models to inspect a healthcare firm's current and future needs.
Conclusion
Health and life science organizations can unlock new opportunities by implementing data analytics in healthcare, but addressing technical and administrative challenges takes priority. You must transfer conventional EHR archives to modern cloud computing ecosystems for better insight exploration. Otherwise, your competitors in the healthcare industry who quickly adopt new technologies will surpass you.
Cybersecurity woes and legal risks might discourage some stakeholders from embracing healthcare analytics. As a result, employing data protection and governance compliance strategies will be essential.
Likewise, preparing for data-led workflows will be crucial for healthcare stakeholders seeking consistent business growth and excellent patient recovery outcomes.