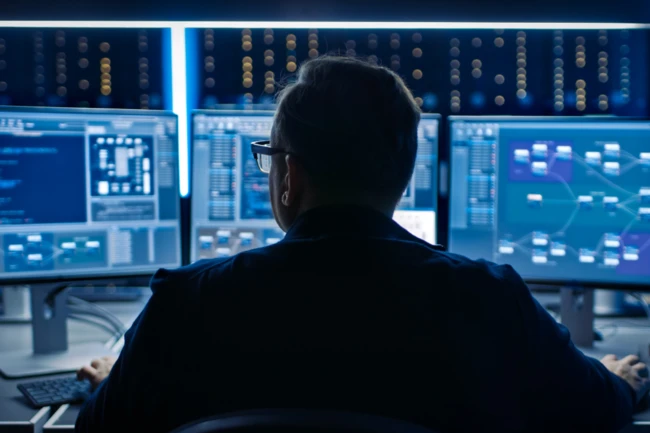
By Chuck Everette, Director of Cybersecurity Advocacy at Deep Instinct
It has been hailed as a transformational technology for cybersecurity. Yet machine learning (ML) is also a dangerous weapon when employed by malicious threat actors and can be a perilous tool when used against common business security. Industries across the world have experienced a dramatic rise in the number of cyberattacks, with reports of new breaches and ransomware attacks making headlines at a worrying frequency. ML is no longer a solution for tackling advancing cybersecurity threats - but it’s now the tool of choice to bypass and break into environments that we thought were protected.
In November 2021, we predicted that attackers will be using machine learning and adversarial AI within 18 months. This prediction still stands - which means that in a year’s time we expect to see threat actors armed with adversarial AI. Organisations are strongly advised to prepare now because this technology is already being weaponised by nation state threat actors, and now is in the hands of common criminals. There is an arms race underway, and staying neutral is not an option.
How Do Attackers Use Machine Learning?
The machine learning technique that is of particular concern to us is Adversarial AI, which evades detection by exploiting the analytic and decision-making powers of established machine learning-based security tools. Adversarial AI is capable of convincing security systems that it is benign. Like a wolf in sheep’s clothing, adversarial AI can sneak past defences and wreak havoc inside the network while staying relatively hidden and flying under the radar.
There are three main types of adversarial AI attacks.
AI-based Cyberattacks – We are already seeing this technique in the wild. It is not yet at scale, but we predict that it is only a matter of time before it becomes widespread. AI-based cyberattacks involve threat actors deploying malware that uses ML algorithms in its attack logic. ML-powered malware can automate activities that once required manual human guidance. This makes the malware fast, aggressive and capable of acting independently from the threat actor that deployed it.
AI-facilitated Cyberattacks – This involves deploying malware on an endpoint and using AI-based algorithms on the attacker's client-side server, where it can automatically sift through data and identities at high speed to orchestrate and optimise further automated attacks. An example would be an info-stealer malware that can exfiltrate a large dataset of personal information using an AI algorithm to discover and classify valuable personal data such as passwords or credit card numbers.
Adversarial Learning – Traditional ML tools must be trained with data sets in order to identify patterns. But threat actors can use false data sets and make the algorithm to classify data incorrectly - which is called adversarial learning or “Data Poisoning”. Right now, this is essentially a theoretical threat. But if adversarial learning becomes more widespread, it will render ML-powered security systems totally redundant because it will teach them to classify malware as harmless. Although these threats are serious and real, they are not yet endemic out in the wild. There is still time to mount a defence, and the technology to do this is already on the market. Swift action today will lay the groundwork for better security in the future.
The Problem With Machine Learning in Cybersecurity
Security systems that use ML are not the solution to the rise in adversarial AI attacks we expect to see in the coming years. Basic ML-based tools over-protect by slowing business operations and hitting defenders with a constant tsunami of false positives commonly referred to as “alert fatigue”. Yet they also under-protect and lack the speed, precision and scalability to predict and prevent unknown malware and zero-day threats that sophisticated threat actors are employing at an ever-increasing frequency.
ML solutions are trained to identify patterns, which is achieved by feature engineering in which the tool is manually fed pre-labeled datasets and taught to distinguish between benign and malicious activity. ML is often used to analyse threat data and tackle routinely expected threats, freeing up security teams' time and allowing them to focus on complex work that requires human attention. However, ML is trained on limited datasets that quickly become outdated. When confronted with a new or unknown threat, it crumbles. It is impossible to train legacy ML to recognise a threat that has never been seen before.
Malware is now able to execute before ML even notices it is a threat, with the fastest ransomware now encrypting files within 15 seconds of activation. Feeding ML false data using adversarial AI also teaches them to ignore threats and misclassify malware as benign. Attackers that use ML will soon be running rings around defenders that use ML in their own cybersecurity defense.
The Case for Deep Learning
To cope with ML-based attacks and close the cybersecurity vulnerabilities it creates, organisations should deploy a more robust technology like the advanced AI of deep learning. This technology is now moving into the mainstream, with Tesla, Google and Amazon investing heavily in the space to power applications such as medical research, self-driving vehicles, or the deep analysis of user behaviour.
Deep learning employs powerful neural networks inspired by the human brain. The tools used can train themselves independently so they can process much larger datasets than traditional ML tools that require manual input. During the training process, deep learning tools are left alone to process large amounts of raw data, which is then classified as benign or malicious much like the human brain would. Deep learning when done properly can also be naturally resistant to “data poisoning” unlike legacy machine learning that is extremely vulnerable to this type of attack and bypass.
This enhanced training methodology allows deep learning to recognise unknown threats and therefore cuts the vast number of false positives experienced by so many security teams. It is able to identify more complex patterns than ML at much higher speeds, with the fastest example of this game-changing tech capable of detecting and blocking malware in just 20 milliseconds. Advanced solutions even can now identify advanced malware before it enters the IT environment. Deep learning is so fast that it allows organisations to move beyond simply mitigating attacks and change their mindset to prevention - which is precisely where we need to be.
Adversaries are now starting to use ML and adversarial AI at the same time as traditional ML-based security tools usefulness fades and fails to keep up with today’s sophisticated attack vectors. This is a dangerous moment in cybersecurity which requires a fundamental shift in thinking. Deep learning is the solution to the problems caused by ML - which truly will be one of the most significant threats of tomorrow. Deep learning is the future of cyber security.