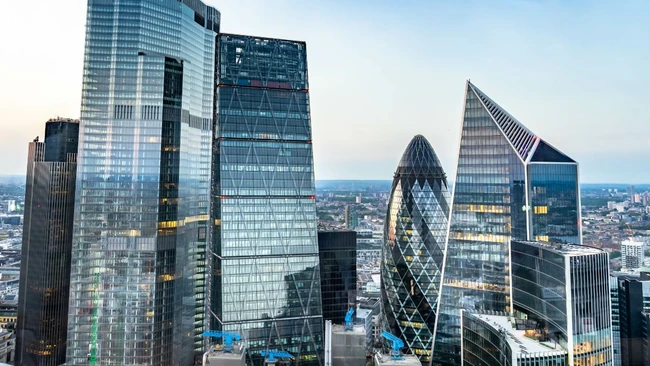
In today's data-driven world, real-time data and analytics have become indispensable for making informed decisions and providing personalised customer experiences. Indeed, many organisations have been on a journey towards real-time capability for years, and post-web startups mostly begin life as ‘real-time natives’.
And, with the ‘mobile first’ revolution continuing to shape consumer expectations to the extent that real-time experiences are demanded in a wide range of digital communications and transactions, the corporate race to provide customers with real-time experiences is only intensifying.
However, there are potential risks associated with relying solely on real-time data that centre primarily around matters of accuracy and interpretation.
In this article, we’ll look at why real-time data and analytics are prone to inaccuracy and how issues arise with interpreting both. We’ll then move on to describe the tools that are helping businesses overcome these persistent challenges.
Inaccurate Real-Time Data and Analytic Pose Real Risks
When incorrect or outdated information is used to generate the likes of status updates or personalised offers, the subsequent service delivered to customers will inevitably be misguided. Although real-time data streams typically improve the availability and velocity of an organisation’s data, where inaccuracies lead to such misguided services, customer trust is undermined, and brand reputation is tarnished.
Aside from inaccurate data, there is also the risk that organisations leverage data that they aren’t supposed to. When a customer is presented with offers based on information they didn’t explicitly consent to share, the question: "How does this company know that information about me?" is asked with suspicion, even anger. Hardly an ideal place to begin nurturing a positive customer relationship.
Erroneous Interpretation and the ‘hallucination’ phenomenon
Presenting another serious risk to organisations are conclusions drawn from incomplete information. The enhanced availability and speed of real-time data are nullified when the full picture is absent and can lead to organisations making hurried decisions that fail to align with specific circumstances.
Although flawed interpretation of data can often be chalked up to human error, where the data is incomplete or inadequate to begin with, businesses are rendered helpless in the face of inescapable suboptimal outcomes.
As the reliance on AI increases, incomplete data and human error are joined by another issue of the most modern variety. Generative AI, such as ChatGPT-style chatbots, are known to ‘hallucinate’ when they don’t have enough information, causing them to invent information as a means of plugging any gaps.
The Tools Available to Make Real-Time Data Work
Despite real-time data streams improving the availability and velocity of an organization’s data, they have led many to move from highly normalised, well-structured data warehouses to unstructured data lakes.
Accordingly, the challenge has become less about how to collect more data and more about how to effectively harness existing data. Many organisations have adapted to this challenge by collecting large volumes of data and utilising it for offline analysis. However, problems remain with filtering data, synthesising siloes, ensuring data is current and high quality, deriving accurate insights, and injecting those insights into real-time customer experiences and automated business processes.
The solution requires data sources to be seamlessly integrated with those applications responsible for executing an organisation’s core processes and providing vital customer experiences. Here, real-time data streams can provide the flow of data, but additional capabilities, such as iPaaS, API Management, Data Governance, and AI, are also required to ensure systems function effectively and efficiently.
Moreover, streams must be complemented with data governance tooling to ensure the data’s quality and completeness. Workflow tooling is also needed to provide appropriate filtering and contextualisation for enabling accurate insights and reducing the risk of arriving at misleading conclusions. Elsewhere, integration tools play a crucial role in real-time data analytics by facilitating seamless data flow across various systems and platforms, ensuring the right endpoints are reached, and minimising the overall risk associated with relying on real-time analytics.
Are organisations' infrastructures ready for real-time deployments?
At present, most organisations’ infrastructures are not quite ready for real-time deployments, but the fundamental building blocks are there. Further progress will come from two worlds that are currently converging in enterprise IT, that of the user-facing application, which is inherently real-time, and that of analytics, which is more batch-driven to handle the volume of enterprise data.
Big data technology is driving this convergence, having evolved to handle huge volumes of data at speed and scale. Moreover, it has combined with the explosion of next-generation AI, which resides in the analytics world but whose value is realised in the application world and looks set to further accelerate the convergence between the two.
As real-time data analytics continue to evolve, it’s imperative for organisations to recognise and address the potential risks. By embracing data governance, workflow tools, and integration strategies, organisations can harness the power of real-time data while mitigating the risks of inaccuracy, incomplete information, and erosion of customer trust.