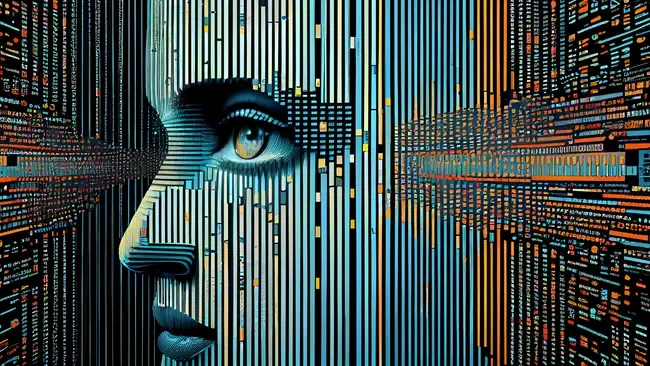
Generative AI is rapidly becoming a game-changer in the business world, with an impressive 35% of companies having already adopted it, according to IBM's Global AI Adoption Index of 2022. This fast-growing field transforms businesses' operations, allowing them to tackle complex challenges easily.
Generative AI tools like OpenAI and other ChatGPT alternatives can sift through massive amounts of data, providing unique insights that conventional methods often need help to deliver promptly.
The impact of generative AI on businesses is far-reaching, spanning from automating content creation to streamlining supply chains and enhancing customer service. By harnessing the power of machine learning and natural language processing, generative AI tools equip companies with the knowledge they need to make better decisions, optimize their processes, and ultimately boost their bottom line.
Defining Generative AI
Generative AI is a subset of artificial intelligence that focuses on creating new data, content, or designs by learning from existing data. Unlike traditional AI models that make predictions based on input data, generative AI models can generate entirely new outputs by understanding the underlying patterns and structures in the data.
The rise of AI in business transformation
Artificial intelligence has become a driving force behind the digital transformation of businesses across various industries. AI-powered technologies enable companies to streamline operations, improve decision-making, and enhance customer experiences. Generative AI, in particular, offers unique opportunities for businesses to innovate and stay ahead of the competition.
Application of Generative AI across industries
In today's competitive landscape, businesses worldwide are exploring ways to harness generative AI to meet their needs and gain an advantage. Here are five practical applications of generative AI that can benefit your organization.
1. Marketing content creation
Marketing is essential for raising awareness and forging emotional connections with potential customers. Generative AI streamlines content creation, enabling marketers to focus on creative concepts. Tools like Jasper AI, Writer AI, DesignerBot by Beautiful.ai, Runway ML, and Mutiny optimize content generation, allowing marketers to fine-tune their strategies.
2. Sales & sales development
While product-led growth is gaining momentum, personalized sales strategies remain crucial. Generative AI allows sales teams to balance quality and quantity without sacrificing efficiency. SellScale and Outreach's Smart Email Assist leverage AI to customize outreach and automate email copy, freeing up time for personalization and editing.
3. Customer support automation
Generative AI has the potential to transform customer support by automating responses while maintaining quality. Customer support is vital for fostering loyalty, but resource and staffing limitations can hinder performance. Tools like Forethought and Cohere enable businesses to build customer support automation or conversational AI tools, improving efficiency and customer satisfaction.
4. Natural language coding & app development
Generative AI redefines application development by allowing developers to use natural language instead of traditional coding languages. GitHub Copilot and Arcwise assist developers with code generation for various languages and even create formulas and macros for spreadsheets. Generative AI accelerates application development and lowers barriers to entry for new developers.
5. Data privacy & synthetic data
As data privacy becomes increasingly critical, businesses must adhere to regulations like GDPR and CCPA. Generative AI can help by generating artificial data that preserves privacy while maintaining natural characteristics for testing and training machine learning models. Tools like Mostly.ai, Tonic.ai, and Private AI enable businesses to maintain data security while using data for production workloads.
Best-suited generative models for businesses
1. GANs (Generative Adversarial Networks)
Image synthesis, data augmentation, and style transfer are just a few of the applications of GANs. These powerful models can help businesses create realistic images for marketing materials, improve data quality, and develop unique visual styles for their brands.
2. VAEs (Variational Autoencoders)
Anomaly detection, denoising, and feature extraction are some of the uses of VAEs. These models can help businesses identify and address potential issues in their data, improving overall data quality and enabling more accurate predictions.
3. RNNs (Recurrent Neural Networks)
Text generation, speech synthesis, and time series prediction are among the applications of RNNs. By leveraging RNNs, businesses can generate natural-sounding text, create a realistic speech for virtual assistants, and forecast future trends more accurately.
Challenges of Generative AI Implementation
1. Data quality and availability
High-quality data is crucial for the success of generative AI models. However, obtaining and maintaining a large dataset can be challenging for businesses, particularly when dealing with sensitive or proprietary information.
2. Ethical concerns and biases
Generative AI raises several ethical concerns, such as the potential for AI-generated content to spread misinformation or perpetuate existing biases. Businesses must carefully consider these issues when implementing generative AI technologies.
3. Integration with existing systems
Integrating generative AI models into business processes and existing systems can require significant technical expertise. Companies must ensure that their infrastructure can support these new technologies and that their teams have the necessary skills to implement and manage them.
4. Skills gap and workforce readiness
The rapid advancement of AI technologies has created a skills gap in the workforce, with many organizations needing help finding employees with the necessary expertise in AI and generative models. To successfully implement generative AI, businesses must invest in upskilling their workforce and fostering a culture of continuous learning.
How can businesses implement Generative AI in their business?
As one of the most rapidly evolving domains in artificial intelligence, generative AI, powered by large language models (LLMs) like ChatGPT, has garnered significant attention in the business world. The excitement around generative AI is genuinely warranted, as advancements in LLMs have made it more potent and applicable across various industries. With its remarkable capacity to generate original content, such as music, images, or text, generative AI is poised to transform sectors and reshape how we live and work.
This excitement has made business executives ponder a crucial question: “How can I effectively implement generative AI in my organization?”
Let’s try and answer this question, shall we?
Implementing Generative AI in business requires a thoughtful approach, considering each organization's unique challenges and opportunities. Here are some creative yet feasible ways companies can integrate Generative AI into their operations:
1. Establish a clear vision and strategy
Identify specific use cases for Generative AI within your organization. Set realistic goals and prioritize projects that can significantly impact efficiency, cost reduction, or revenue generation.
For example, a fashion e-commerce business identifies that Generative AI can create personalized product recommendations, automate customer support, and generate targeted email campaigns. Based on this assessment, they must set a realistic goal of increasing their revenue by 15% and reducing customer support costs by 10% within a year.
2. Start with pilot projects
You can start by implementing Generative AI in a small, manageable area of your business to learn and adapt before scaling up. This could include generating marketing copy, automating customer support, or optimizing logistics and supply chain processes.
For example, a logistics company begins by implementing Generative AI to optimize delivery routes for a specific region. Before expanding the project to other areas, they test the system's effectiveness in reducing fuel costs and delivery times.
3. Collaborate with AI experts
Partner with AI research organizations or hire in-house experts to ensure your Generative AI implementation is well-informed and effective. This can help identify the tools and technologies for your specific use cases.
For example, an automotive manufacturer partners with a leading AI research organization to develop a Generative AI model that can simulate and optimize vehicle designs, enabling faster, more efficient product development.
4. Invest in data infrastructure
Generative AI models rely on large amounts of data to function effectively. Please make sure your business has a robust data infrastructure that can handle the volume, variety, and velocity of the data needed for Generative AI.
For example, a healthcare organization upgrades it's data storage and processing capabilities to handle the massive amounts of patient data required for training and deploying Generative AI models for diagnosis and treatment planning.
5. Develop a data governance framework
Establish policies and procedures for collecting, storing, and using data ethically and securely. This will help mitigate potential risks associated with data privacy, security, and bias in AI-generated outputs.
For example, a financial services firm establishes guidelines for securely collecting and storing customer data in Generative AI models, ensuring compliance with regulations such as GDPR and CCPA.
6. Focus on employee training and development
Educate your workforce on the benefits and applications of Generative AI. Offer training programs to help employees develop the skills to collaborate effectively with AI systems.
For example, a marketing agency offers workshops and training sessions for its staff to learn how to use Generative AI tools for content creation, design, and strategy optimization.
7. Foster a culture of experimentation and innovation
Encourage employees to explore new ideas and approaches, incorporating Generative AI into their daily tasks. Reward those who find creative ways to apply AI to their work, driving organizational innovation.
For example, a software development company holds regular hackathons, encouraging employees to explore new ways to use generative AI to improve their products and streamline development processes.
8. Monitor and measure success
Track the performance of Generative AI projects and identify areas for improvement. Use analytics to evaluate the impact of AI on your business and adjust your strategy accordingly. For example, a retail business tracks the performance of its AI-generated marketing campaigns, analyzing metrics such as conversion rates and customer engagement to refine its strategy and optimize future campaigns.
9. Ensure ethical and responsible AI usage
Be mindful of the potential ethical concerns surrounding Generative AI, such as biases and misinformation. Establish guidelines and best practices for responsible AI usage and monitor its impact on society.
For example, a news organization develops guidelines for using Generative AI in journalism, addressing potential issues such as AI-generated misinformation and biases in AI-generated content.
10. Keep up with the latest advancements
Please stay tuned for the latest developments in Generative AI research and applications. Continuously refine your strategy and adopt new technologies to maintain a competitive edge.
For example, a consulting firm regularly attends AI conferences, subscribes to industry publications, and participates in online forums to stay informed about the latest developments in Generative AI, adapting their services and strategies accordingly.
Concluding thoughts
As Generative AI continues to evolve and improve, organizations that embrace these cutting-edge technologies will be better positioned to innovate, stay ahead of the competition, and ultimately enhance their overall performance.