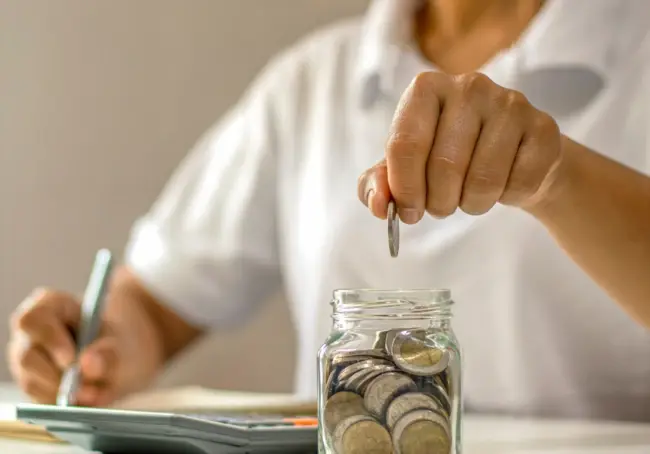
Big data analysts will ask companies for extensive data warehousing infrastructure and efficient extract-transform-load (ETL) pipelines. Meanwhile, cloud platforms charge their clients according to the operational scale or consumed computing resources. Each data operation will need a specific upfront investment. However, this post will demystify how to minimize your data collection and analysis cost.
What is Data Analytics?
Data analytics combines computer technologies and mathematical modeling methods to get strategically-beneficial insights from a corporation’s core databases. Today’s businesses employ data analytics services that can report industry trends and consumer behavior through complex statistical models. Moreover, automated data sorting has become possible thanks to machine learning (ML).
Brands utilize the furnished reporting insights to track the changes in their business model’s strengths and problems. After all, leaving inefficient processes unaddressed will adversely affect their quarterly targets, investor returns, and future expansion plans.
A global enterprise will process multi-lingual data. Therefore, ML-enhanced context detection will matter to it. However, a local business with a domestic-only market scope will request a more straightforward technological tool. These cases also highlight that data analysis and collection costs will vary from company to company.
How Can a Company Minimize the Cost of Data Collection and Analysis?
Approach 1 – Invest in Goal-Driven Data Processes
Relevance is the key to cost reduction across all data solutions. Ask your team how gathering data on a performance or customer relations metric will benefit your business model. Remember, you want data analysis as an instrument that solves current business problems and discovers growth opportunities.
The following cases can assist you in determining the company’s immediate data needs.
Goal 1 – Your Goal is to Consolidate Historical Performance Data
Descriptive analytics specializes in reporting how your sales, customer retention rate, employee productivity, and supply chain resilience have evolved. Managers must invest in descriptive analytics if they want to document the trends in a firm’s historical ups and downs. It will help bring down the billing liabilities.
Goal 2 – You Intend to Discover Why a Project Failed to Meet the Stakeholders’ Expectations
Hire diagnostic analysts. They build upon descriptive analytics reports while facilitating root-cause examination. Managers can use diagnostic analysis to answer queries similar to the following examples.
-
Did a department miss their sales target?
-
Was the marketing effort, customer service, or pricing strategy insufficient?
-
What might have happened to your competitor’s sales in the same quarter?
-
What can you do if you want to prevent identical underperformance this quarter?
Goal 3 – You Want to Inspect Potential Challenges and Opportunities Concerning an Idea
Risk estimation relies on qualitative prediction models aided by ML algorithms, and prescriptive analysts professionally use them. Predictive and prescriptive analysts will help team leaders recognize the idea implementation problems before they arise. Therefore, the company can minimize data collection and analysis costs by focusing on predictive analytics.
Approach 2 – Embrace a Unified, Optimized, and Lean Data Ecosystem
Poor data quality leads to purposeless resource consumption. For example, maintaining separate databases describing one performance metric will force you to spend twice on data storage and network bandwidth.
Eliminating redundant data to increase efficiency and reduce data acquisition costs (DACs) is at the core of a lean data management philosophy. Some proponents of lead data also prioritize adequate data governance and privacy-respecting technology integrations.
Consider how many organizations ask employees to submit near-identical data throughout a financial year. However, a well-optimized data retrieval system will ensure nobody inputs or demands a reporting metric in multiple places a dozen times. That is why lean systems’ “one-time data” principle is incredible.
Although the lean approach promises waste minimization, an established organization will need more time to migrate its legacy systems. Moreover, consolidating distinct file management and formatting systems will necessitate compatibility troubleshooting. Otherwise, lean data analysis is one of the best approaches to minimize the data collection cost.
Conclusion
Each company has developed in-house data management protocols. Nevertheless, they allocate significant resources to maintaining and securing the underlying data ecosystem. They have suffered from double billing due to data duplication and compatibility errors. And this situation makes them think all data operations cost a fortune.
Conventional data processing methods are inefficient. Thankfully, novel approaches that fulfill enterprise data needs are also present. You can discontinue collecting irrelevant “noisy” data to minimize the data analysis cost.
Besides, integrating a lean data philosophy while optimizing and consolidating databases is a practical solution. Select the technology, professionals, and data strategies using these methods to regulate the financial burden of data operations.