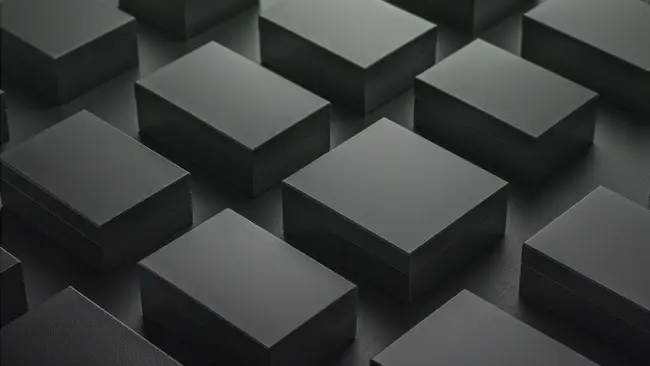
Nothing has gripped Silicon Valley quite like artificial intelligence (AI) over the past few years. Since the launch of OpenAI’s ChatGPT in November 2022, the technology has had the tech industry in a chokehold – dominating discussions across the space and fuelling investments in AI projects from companies big and small.
There’s an AI model for writing content, creating art, and even solving complex mathematical problems. But for all the impressive things AI can do, one question casts a shadow of doubt over its development: How does AI make its decisions?
This problem, known as the AI black box, has left experts concerned about the future of society as AI infringes on every aspect of it.
This article delves deep into the black box AI problem, exploring what it is, why experts are concerned, and how it will impact the future of AI development.
What is the Black box AI problem?
The AI black box problem refers to the lack of transparency of AI systems whose internal mechanics are hidden from those who develop them. AI models take in inputs and produce outputs, but the logic and data used to reach those results are not accessible, making it difficult — or even impossible — to fully see how they make the decisions they make.
This creates a ‘black box’ in AI that humans can't access.
When an AI system makes a decision with significant consequences (e.g., denying a loan or recommending a medical treatment), it's crucial to understand how it reached that decision.
But deep learning models don't follow a straightforward linear path to reach their conclusions. Even developers who create these models may not fully understand how they work, making it difficult to explain their decisions to others.
This makes it difficult to trace the reasoning behind its decision or understand how it works down to the granular level.
If we don’t understand how AI makes a decision, how can we be sure the decision it makes is ethical and correct? That’s the question experts are asking as AI becomes increasingly prevalent across all sectors of society.
Why is the black box AI problem so dangerous?
Because of the black box, it's almost impossible to pinpoint the source of the error and fix it when an AI system makes a mistake.
The complex inner workings of the algorithm can be like an impenetrable black box, making it difficult to diagnose and correct the issue. This can lead to persistent errors and potentially dangerous consequences as AI becomes more embedded into our society.
Let’s say for example an autonomous vehicle makes a wrong turn and ends up in a serious accident. The complex inner workings of the algorithm that decided to make the turn are impossible to decipher, and its developers can’t diagnose and correct the issue.
Another key issue is AI bias. If an AI system is trained on biased data, it can perpetuate those biases in its decisions. This can lead to discriminatory outcomes, such as denying loans to people of colour or recommending harsher sentences for minorities.
We saw this with Google’s large language model (LLM), Gemini, last month. The model’s AI image generator appeared to have a bias towards minority groups to the extent that it would change the ethnicity of the celebrities and figures it was prompted to display.
Due to the complex nature of deep learning, it's sometimes impossible to predict all the potential consequences of an AI system's decisions. And since Google didn’t understand why the model was portraying this bias, Google was forced to prevent Gemini from generating images altogether.
If people don't understand how AI systems work, they are less likely to trust them. It can lead to unfairness, lack of accountability, and unforeseen consequences, hindering the potential benefits of AI and posing significant risks to society as a whole.
Can the AI black box problem be fixed?
Completely "fixing" the AI black box problem might not be entirely possible, as some level of complexity will always be inherent in advanced AI models. However, we’re making significant progress in developing techniques to improve transparency and interpretability in AI.
One example of this is the rise of explainable AI (XAI), a field focused on designing AI algorithms that can provide clear explanations for their decisions.
XAI involves identifying the specific features or inputs that the AI model relies on most heavily to make its decisions. It then generates explanations for individual predictions by creating simpler models that mimic the behaviour of the original AI model in a specific context.
In some cases, simply using less complex models is another way of making AI more transparent even if it comes at the expense of some accuracy. This can be particularly useful in high-stakes applications where understanding the reasoning behind decisions is crucial.
Combining AI with human expertise is also crucial to making sure AI decisions are made with oversight and understanding. Humans can provide context, set boundaries, and intervene when necessary, making the overall system more transparent and accountable.
The future of black box AI
The black box problem is a serious challenge that AI developers will need to overcome to ensure that AI development remains responsible and ethical.
As the concerns about the black box problem grow, there will likely be a greater emphasis on developing XAI techniques to make AI models more transparent and understandable. Developers will need to combine black box models with simpler, more interpretable models will to strike a balance between accuracy with transparency.
At the same time, developing clear regulations and ethical guidelines for AI development and deployment can help mitigate the risks associated with black box algorithms.
The future of AI development will likely involve a continued effort to address the black box problem and ensure that AI systems are transparent, accountable, and beneficial to society.
AI cannot progress until the black box is opened.